Decoding User Behavior: How AI Interprets Website Interactions
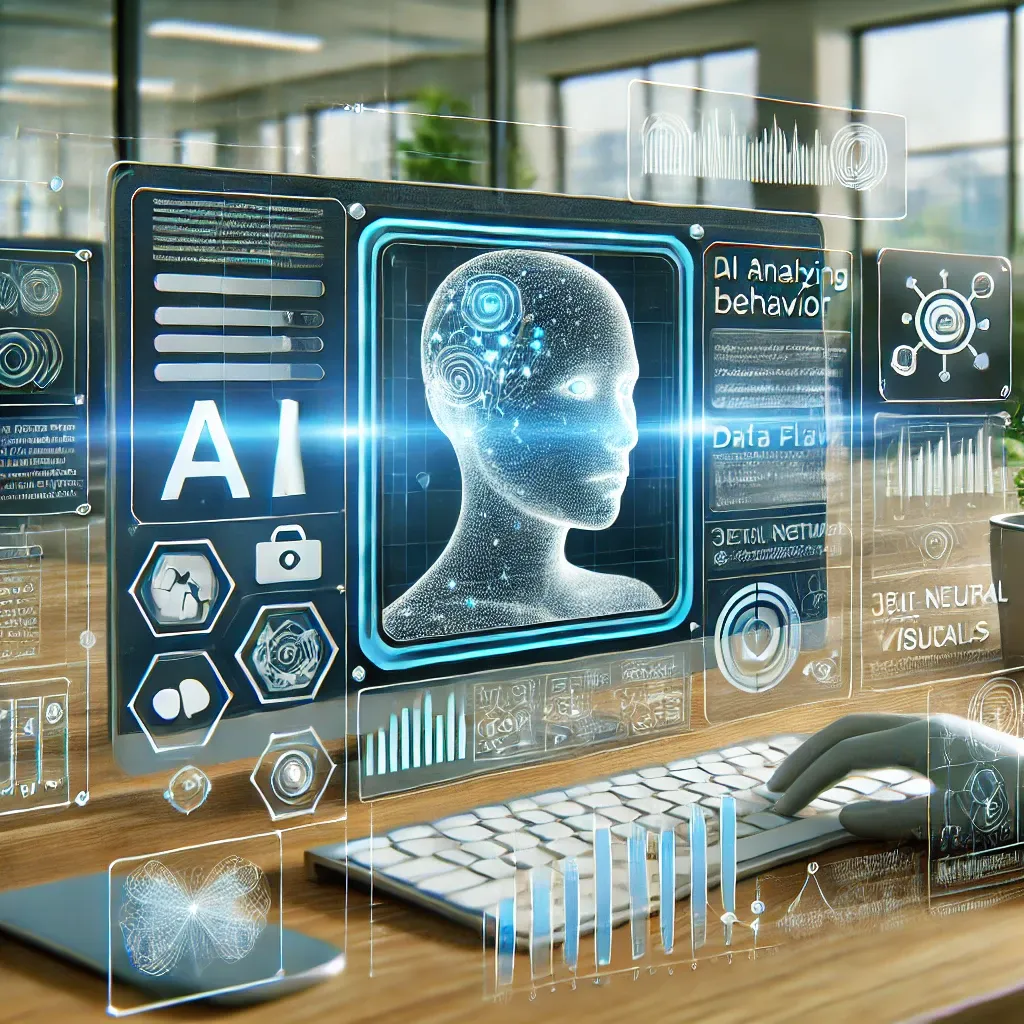
In the digital age, understanding user behavior is crucial for businesses to thrive. Artificial Intelligence (AI) has revolutionized how we interpret and act upon user interactions on websites. This blog post delves into the intricate ways AI decodes user behavior, drawing meaningful conclusions about preferences and intentions. Let's explore the fascinating world of AI-powered user behavior analysis.
The Foundations of AI-Driven Behavior Analysis
Before we dive into specific techniques, it's essential to understand the foundational elements that make AI-driven behavior analysis possible:
- Data Collection: AI systems continuously gather vast amounts of data from various touchpoints on a website.
- Machine Learning Algorithms: These algorithms process the collected data, identifying patterns and correlations.
- Real-Time Processing: Modern AI systems can analyze data in real-time, allowing for immediate insights and actions.
- Contextual Understanding: AI doesn't just look at isolated actions but considers the context in which these actions occur.
Now, let's explore how AI decodes different aspects of user behavior.
1. Click Pattern Analysis
AI goes beyond simple click tracking to understand the intent and emotion behind user clicks.
How It Works:
- Sequence Analysis: AI examines the order of clicks to understand user journeys.
- Timing Intervals: The time between clicks can indicate user engagement or confusion.
- Mouse Movement: AI tracks not just clicks, but also how users move their cursors.
Example:
An e-commerce site uses AI to analyze click patterns. It notices that users often click back and forth between product pages before making a purchase. The AI interprets this as comparison behavior and automatically implements a side-by-side comparison feature, resulting in a 15% increase in conversion rates.
2. Session Duration and Page Time Analysis
AI interprets how users spend their time on a website to gauge interest and engagement.
Key Factors:
- Time on Page: AI considers not just how long a user stays on a page, but also what content is on that page.
- Scroll Depth: How far users scroll can indicate content relevance.
- Interaction Frequency: The number of interactions within a given time frame.
Example:
A news website's AI notices that users spend an average of 5 minutes on long-form articles but only 30 seconds on shorter pieces. However, the AI also detects that users who spend 1-2 minutes on shorter articles are more likely to share them on social media. This insight leads to a content strategy that balances depth with shareability, resulting in a 25% increase in overall engagement.
3. Navigation Path Analysis
AI examines the routes users take through a website to understand their goals and pain points.
What AI Looks For:
- Common Paths: Frequently taken routes that might indicate user preferences.
- Abandonment Points: Where users often leave the site.
- Unexpected Detours: Unusual navigation patterns that might signify confusion or exploration.
Example:
An online learning platform's AI analyzes navigation paths and notices that users who visit the "course syllabus" page before enrolling have a 50% higher completion rate. The AI automatically adjusts the website layout to prominently feature syllabus links, resulting in both higher enrollment and completion rates.
4. Search Query Interpretation
AI doesn't just log search queries; it interprets the intent and context behind them.
Advanced Techniques:
- Natural Language Processing (NLP): Understanding the nuances of human language.
- Query Sequence Analysis: Looking at the series of searches a user performs.
- Context-Aware Interpretation: Considering factors like user location or time of day.
Example:
An e-commerce site's AI notices a trend of users searching for "blue flower vase" but clicking on purple vases in the results. The AI interprets this as a mismatch between color preference and available inventory. It automatically adjusts the product recommendations to feature more blue vases and suggests purple as an alternative, leading to a 30% increase in vase sales.
5. Form Interaction Analysis
AI examines how users interact with forms to optimize the user experience and increase completions.
What AI Monitors:
- Field Order: The sequence in which users fill out form fields.
- Hesitation Time: How long users pause before filling out specific fields.
- Error Rates: Which fields most often cause validation errors.
Example:
A SaaS company's AI analyzes form interactions on their sign-up page. It notices that users often hesitate on the "Company Size" field and frequently abandon the form at this point. The AI tests different versions of this field (e.g., a dropdown vs. radio buttons) and finds that radio buttons with broad categories reduce abandonment by 40%.
6. Content Engagement Analysis
AI interprets how users interact with different types of content to understand preferences and optimize engagement.
Engagement Metrics:
- View Time: How long users spend with different content types.
- Interaction Rate: Likes, comments, shares, and other interactions.
- Return Rate: How often users come back to specific content.
Example:
A fitness app's AI analyzes content engagement and notices that users who watch workout videos in the evening are 60% more likely to use the app the next morning. The AI implements a feature that sends evening notifications with video previews, resulting in a 35% increase in daily active users.
7. Device and Technology Context
AI considers the technical context of user interactions to provide a more nuanced understanding of behavior.
Contextual Factors:
- Device Type: Smartphone, tablet, desktop, etc.
- Screen Size: How the display affects interaction.
- Connection Speed: How performance impacts behavior.
Example:
An airline's website AI notices that mobile users often abandon the booking process on the seat selection page. Further analysis reveals that the seat map is difficult to navigate on smaller screens. The AI automatically simplifies the seat selection for mobile users, reducing mobile booking abandonment by 25%.
8. Emotional Response Interpretation
Advanced AI systems are beginning to interpret emotional responses to website elements.
Techniques:
- Sentiment Analysis: Using NLP to gauge sentiment in user-generated content.
- Facial Expression Analysis: Using computer vision on webcam feeds (with user permission).
- Interaction Intensity: Interpreting the force of clicks or swipes on mobile devices.
Example:
A video streaming service uses AI to analyze facial expressions (with opt-in from users) while they watch trailers. The AI notices that users who smile during comedy trailers are 80% more likely to watch the full movie. This insight is used to personalize movie recommendations, leading to a 20% increase in viewing time.
9. Cross-Session and Cross-Device Behavior Analysis
AI can track and interpret user behavior across multiple sessions and devices for a more holistic understanding.
What AI Examines:
- Return Behavior: How users behave when they come back to the site.
- Cross-Device Journeys: How user behavior changes across devices.
- Long-Term Patterns: Behavioral trends over extended periods.
Example:
A real estate website's AI notices that users often start their search on mobile devices but switch to desktops before contacting agents. The AI implements a feature that allows users to easily save and transfer their search progress between devices, resulting in a 50% increase in lead generation.
Conclusion: The Future of AI-Driven Behavior Interpretation
As AI continues to evolve, we can expect even more sophisticated interpretation of user behavior:
- Predictive Behavior Modeling: AI will not just interpret past behavior but accurately predict future actions.
- Augmented Reality (AR) Interaction Analysis: As AR becomes more prevalent, AI will interpret how users interact with virtual elements in real-world contexts.
- Brain-Computer Interface (BCI) Interpretation: In the long term, AI might directly interpret neural signals to understand user intent and preferences.
The key to successful AI-driven behavior interpretation lies not just in the technology, but in using these insights ethically and effectively. As AI becomes more adept at understanding human behavior, businesses must balance personalization with privacy, and efficiency with user autonomy.
By leveraging AI to decode user behavior, businesses can create more intuitive, engaging, and effective digital experiences. The future of web design and digital marketing lies in understanding users not just as data points, but as individuals with unique preferences, behaviors, and needs. AI is the key to unlocking this understanding at scale.