Decoding User Behavior: How AI Interprets Website Interactions
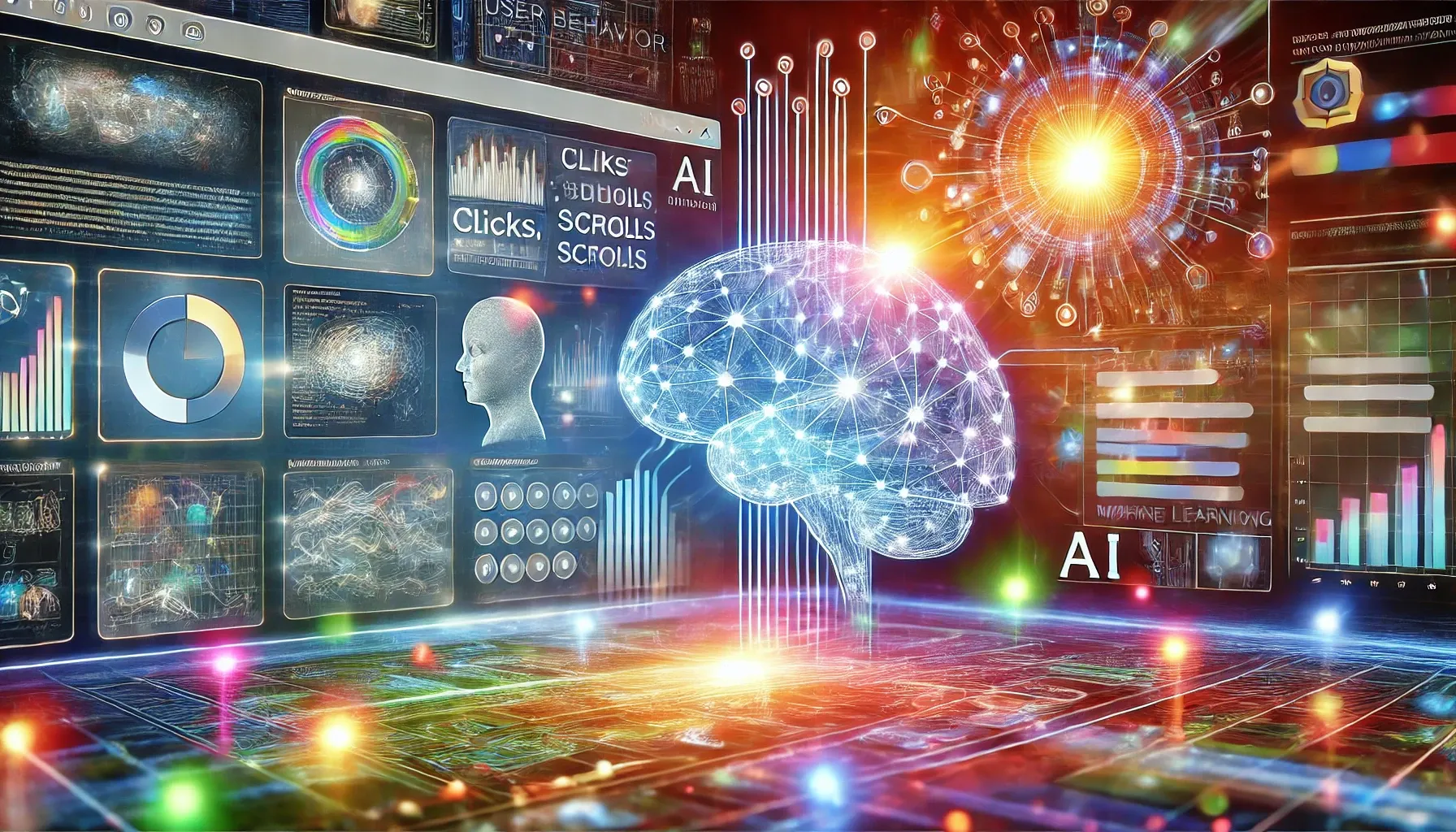
In the ever-evolving digital landscape, understanding how users interact with websites has become crucial for businesses looking to optimize their online presence. Thanks to artificial intelligence, we can now decode complex patterns of user behavior in ways that were previously impossible. This technological advancement has transformed how we understand and respond to user needs in real-time, creating more engaging and effective digital experiences.
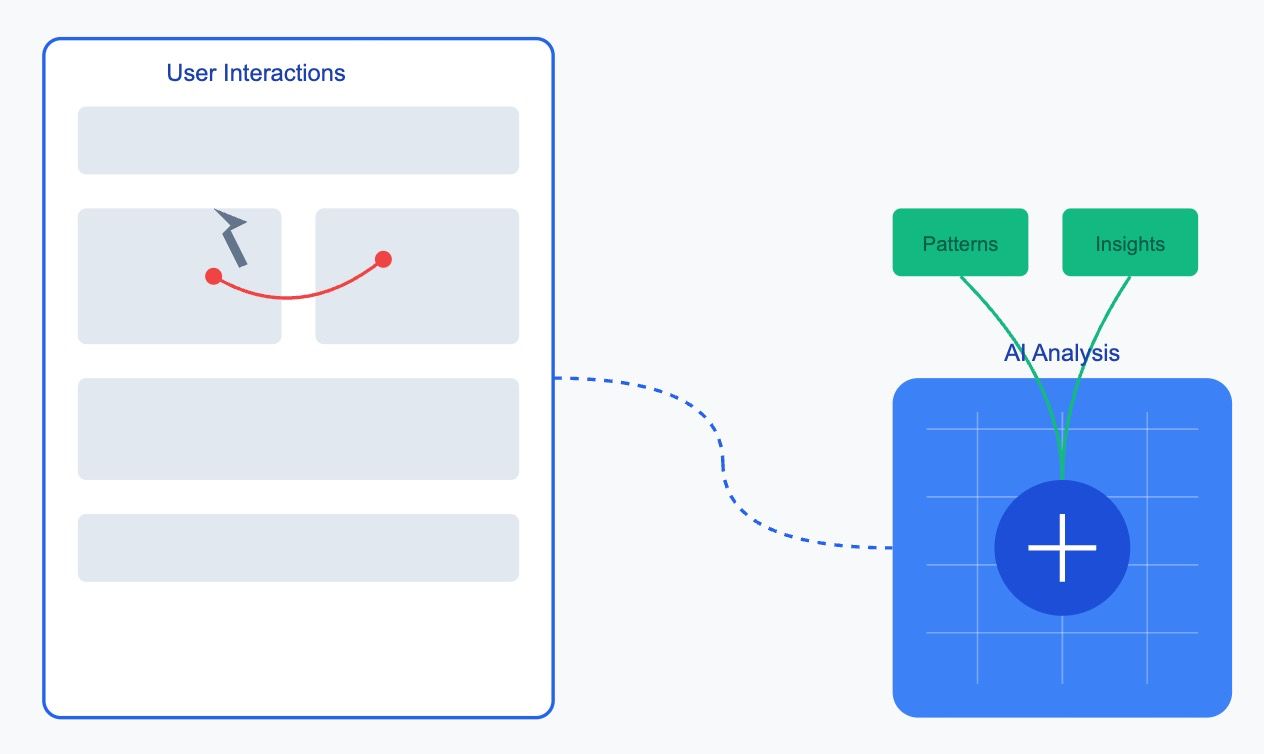
The Digital Body Language
Just as body language reveals our intentions in person, our digital interactions create a unique behavioral signature. Every click, scroll, and pause tells a story about user intent and engagement. AI systems capture and interpret numerous signals that make up this digital body language, creating a comprehensive picture of user behavior.
Mouse Movements and Cursor Patterns
The way users move their mouse across a page reveals valuable insights about their engagement and interest levels. Rapid, direct movements often indicate familiarity with the layout and clear intent, while hesitant, circular movements might suggest uncertainty or confusion. For example, when users repeatedly move their cursor between two options, it often signals comparison behavior or decision-making difficulty.
Interaction Timing and Depth
The time users spend on different page elements provides crucial context about content relevance and user engagement. A quick scan of a page followed by immediate exit might indicate misaligned content with user expectations, while deep engagement with specific sections suggests high relevance. For instance, when users spend several minutes reading product descriptions and reviews before moving to pricing information, it typically indicates serious purchase consideration.
Navigation and Journey Mapping
User pathways through a website tell a story about their goals and decision-making process. AI analyzes these journeys to identify common paths to conversion, potential roadblocks, and opportunities for optimization. Consider an e-commerce site where AI notices that users who visit the size guide before adding items to cart have a 40% higher conversion rate – this insight could lead to more prominent size guide placement.
How AI Connects the Dots
Pattern Recognition
Modern AI systems don't just collect data—they understand context through sophisticated pattern recognition algorithms. These systems analyze millions of interactions to identify meaningful behavioral patterns that indicate user intent, satisfaction, or friction points.
For example, when a user exhibits a pattern of:
- Rapidly scrolling through content
- Returning to the top of the page
- Clicking the navigation menu multiple times
- Finally using the search function
The AI might interpret this sequence as a clear sign of navigation difficulty, prompting improvements to the site's information architecture.
Behavioral Clustering
AI excels at grouping similar behaviors into meaningful segments, creating detailed user personas based on interaction patterns. Here's how different user types typically behave:
Power Users:
- Navigate through sites with precision and speed
- Use keyboard shortcuts and advanced features
- Complete tasks in significantly less time than average
- Often return to the same sections repeatedly
Example: A power user might complete a complex checkout process in under two minutes, using saved preferences and shortcuts.
Casual Browsers:
- Move through content at a leisurely pace
- Often follow recommendation links
- Spend time on images and interactive elements
- Have longer but less focused sessions
Example: These users might spend 15 minutes reading blog posts, following related content links, and browsing without a specific goal.
Goal-Oriented Visitors:
- Follow direct paths to specific information
- Minimal deviation from their intended journey
- Higher interaction with functional elements like forms and filters
- Shorter session times with higher completion rates
Example: A user searching for specific product specifications who navigates directly to technical documentation and exits after finding the information.
Predictive Analysis
AI's ability to learn from historical data enables sophisticated prediction models that can anticipate user behavior and needs. These predictions manifest in several ways:
Conversion Likelihood:
The system analyzes factors such as engagement depth, visit frequency, and interaction patterns to calculate conversion probability. For instance, users who view multiple product images, read reviews, and check shipping information within a single session typically show a 70% higher likelihood of purchasing.
Abandonment Prevention:
AI can identify early warning signs of cart abandonment, such as:
- Rapid switching between cart and other tabs
- Extended inactivity on checkout pages
- Multiple attempts to modify order details
When these patterns are detected, the system can trigger interventions like discount offers or support chat prompts.
Real-World Applications
Dynamic Content Optimization
AI transforms static websites into dynamic environments that adapt to user preferences and behaviors in real-time. Here's how this works in practice:
Content Presentation:
When AI detects a user's preference for video content over text (through engagement metrics), it automatically prioritizes video content in future interactions. For example, if a user consistently watches product demonstration videos, the system might automatically play videos instead of showing static images on product pages.
Navigation Optimization:
Based on user behavior patterns, AI can restructure navigation elements to reduce friction. If analytics show that users frequently search for "returns policy" after viewing product pages, the system might automatically make this link more prominent in the product section.
User Experience Enhancement
AI systems continuously learn from collective user interactions to improve the overall experience:
Process Simplification:
When AI detects common user workflows, it can streamline these processes. For instance, if data shows that users often follow a specific sequence of actions (like checking size guides before adding to cart), the system might combine these steps into a single, smooth interaction.
Friction Point Elimination:
By analyzing where users commonly encounter difficulties, AI can implement automatic solutions. For example, if users frequently abandon forms at a specific field, the system might add helpful tooltips or restructure the form to be more intuitive.
The Future of User Behavior Analysis
The evolution of AI technology is opening new frontiers in behavior analysis:
Emotional Intelligence in AI
Advanced algorithms are now beginning to interpret emotional states from digital behavior patterns. This includes:
- Detecting frustration through rapid, repetitive clicking
- Identifying satisfaction through smooth, purposeful navigation
- Understanding engagement levels through interaction rhythm and flow
- Recognizing confusion through chaotic navigation patterns
For example, if a user exhibits signs of frustration (like rapid clicking or erratic scrolling), an AI system might automatically simplify the interface or offer assistance through a chatbot.
Cross-Device Understanding
Modern AI systems can now create unified user profiles across multiple devices and sessions, providing deeper insights into the complete customer journey. This might reveal that a user researches products on mobile during their commute but completes purchases on desktop in the evening, allowing for optimized experiences on each platform.
Privacy and Ethics
While AI's capabilities in understanding user behavior are powerful, maintaining user trust through responsible data practices is paramount:
Transparent Data Collection:
- Clear communication about what data is collected and why
- Detailed privacy policies written in accessible language
- Regular updates about how data is being used to improve user experience
- Options for users to view and manage their data preferences
Security Measures:
- End-to-end encryption of user data
- Regular security audits and updates
- Strict access controls and monitoring
- Compliance with international data protection regulations
Conclusion
AI's ability to decode user behavior has transformed how we understand and optimize digital experiences. By continuously learning from millions of interactions, AI systems help create more intuitive, responsive, and personalized websites. The key lies not just in collecting data, but in using AI to transform that data into actionable insights that enhance user experience while respecting privacy.
As these technologies continue to evolve, we can expect even more sophisticated understanding of user behavior, leading to truly adaptive digital experiences. The organizations that best leverage these AI capabilities while maintaining user trust will be the ones that succeed in the digital landscape.
The future of web interaction lies in this delicate balance between powerful AI-driven personalization and responsible data stewardship. As we move forward, the focus will increasingly be on creating experiences that are not just smart, but also ethical and user-centric.