Measuring Success: KPIs for AI-Driven Website Optimization
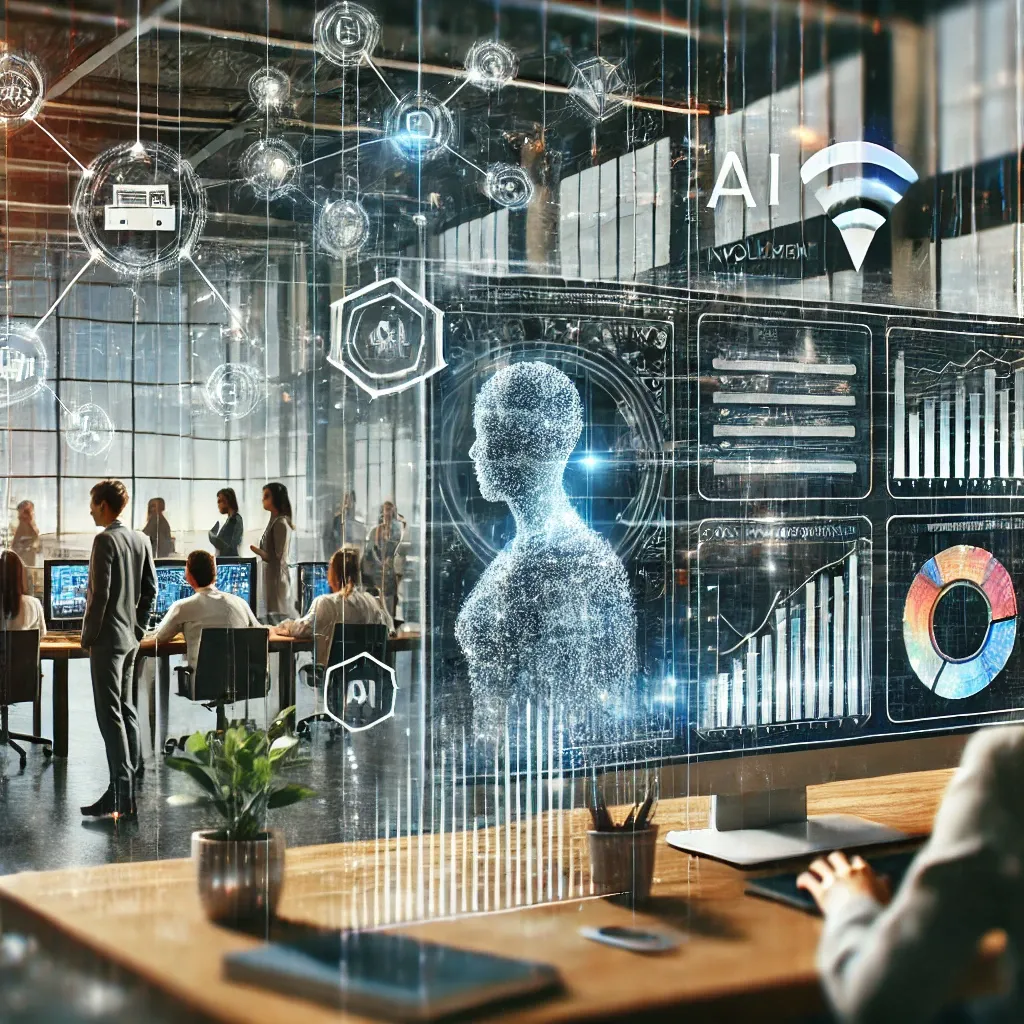
In the era of AI-driven website optimization, measuring success has become both more complex and more insightful. As artificial intelligence and machine learning technologies reshape how we approach website performance, it's crucial to understand the key performance indicators (KPIs) that truly matter. This post will dive deep into the most relevant KPIs for AI-optimized websites, providing you with the knowledge to effectively gauge and improve your site's performance.
The Shift in KPI Landscape
Before we delve into specific KPIs, it's important to understand how AI has changed the landscape of website optimization metrics:
- Real-time vs. Static: Traditional KPIs often relied on periodic reporting. AI-driven optimization allows for real-time tracking and adjustment.
- Predictive vs. Reactive: AI enables predictive analytics, shifting focus from what has happened to what will likely happen.
- Personalized vs. Aggregate: Instead of looking at overall metrics, AI allows us to measure success on a per-user or per-segment basis.
- Multi-dimensional vs. Single-dimensional: AI can process and analyze multiple KPIs simultaneously, providing a more holistic view of performance.
With this shift in mind, let's explore the essential KPIs for AI-driven website optimization.
1. Conversion Rate Optimization (CRO) KPIs
While conversion rate remains a crucial metric, AI brings new dimensions to CRO:
a. Dynamic Conversion Rate
Description: This KPI measures how conversion rates change in real-time as AI makes adjustments to the website.
Example: An e-commerce site uses AI to dynamically adjust product recommendations. The dynamic conversion rate tracks how these changes impact conversions throughout the day, allowing for minute-by-minute optimization.
b. Predictive Conversion Probability
Description: AI models can assign a conversion probability to each user session in real-time.
Example: A B2B software company uses this KPI to identify high-potential leads. If a user's predictive conversion probability exceeds 80%, the AI triggers a chatbot to offer immediate assistance, increasing the chances of conversion.
c. Segment-Specific Conversion Rates
Description: AI can track conversion rates for highly specific user segments.
Example: An online education platform uses AI to create micro-segments based on learning style, career goals, and past behavior. By tracking segment-specific conversion rates, they can tailor course recommendations and UI elements to each segment, dramatically improving overall conversion rates.
2. User Engagement KPIs
AI-driven websites can adapt in real-time to user behavior, making engagement metrics more dynamic and insightful.
a. Adaptive Session Duration
Description: This KPI measures how long users stay on the site as it adapts to their behavior.
Example: A news website uses AI to adjust article recommendations and layout based on user engagement. The adaptive session duration KPI helps them understand how these real-time changes impact the time users spend on the site.
b. AI-Optimized Click-Through Rate (CTR)
Description: This measures the effectiveness of AI-driven content and CTA placements.
Example: An e-commerce site uses AI to dynamically adjust the position and design of "Add to Cart" buttons based on user behavior. The AI-optimized CTR tracks how these adjustments impact click-through rates across different user segments.
c. Engagement Depth Score
Description: An AI-calculated score based on multiple engagement factors like scroll depth, time on page, and interactions.
Example: A SaaS company uses this KPI to measure the quality of engagement on their blog. The AI assigns higher scores to users who not only spend time reading but also interact with embedded calculators or video content, helping to refine the content strategy.
3. Personalization Effectiveness KPIs
AI excels at personalization, and these KPIs help measure its impact:
a. Personalization Uplift
Description: Measures the improvement in key metrics (like conversion rate or average order value) for users receiving personalized experiences versus a control group.
Example: An online retailer uses AI to personalize product recommendations. They measure a 25% increase in average order value for users receiving AI-driven recommendations compared to a control group, quantifying the ROI of their AI investment.
b. AI Recommendation Acceptance Rate
Description: Tracks how often users engage with AI-recommended content or products.
Example: A streaming service uses this KPI to gauge the effectiveness of its AI recommendation engine. A high acceptance rate indicates that the AI is successfully predicting user preferences, while a low rate might suggest need for algorithm refinement.
c. Dynamic Customer Lifetime Value (CLV)
Description: An AI-powered prediction of a customer's long-term value, updated in real-time based on interactions.
Example: An insurance company uses dynamic CLV to adjust their customer service approach in real-time. High CLV customers might be immediately routed to premium support, while the AI simultaneously suggests personalized upsell opportunities.
4. AI Performance KPIs
These KPIs help measure the effectiveness of the AI system itself:
a. Algorithm Confidence Score
Description: A metric indicating how confident the AI is in its decisions or predictions.
Example: A financial services website uses AI to recommend investment products. The confidence score helps determine when to rely on AI recommendations and when to default to standard offerings, ensuring regulatory compliance and customer trust.
b. Learning Rate
Description: Measures how quickly the AI model improves its performance over time.
Example: An e-commerce platform tracks the learning rate of its product recommendation AI. A high learning rate might indicate that the model is quickly adapting to new user behaviors or product trends, while a plateau could suggest the need for model refinement or more training data.
c. AI-Human Collaboration Efficiency
Description: Measures the effectiveness of combined AI and human efforts in website optimization.
Example: A lead generation website tracks how efficiently their marketing team implements and refines AI-generated optimization suggestions. This KPI helps them balance automation with human oversight, ensuring AI remains a tool that enhances rather than replaces human creativity.
5. Technical Performance KPIs
While these KPIs aren't unique to AI-driven sites, they take on new importance in an AI context:
a. Adaptive Page Load Time
Description: Measures how quickly pages load as AI adjusts content and structure in real-time.
Example: A media site uses AI to dynamically adjust page layouts based on user preferences and device capabilities. The adaptive page load time KPI ensures that personalization doesn't come at the cost of speed, critical for user experience and SEO.
b. AI Processing Latency
Description: Tracks the time taken for AI to make and implement decisions.
Example: An online gaming platform uses AI to adjust game difficulty in real-time. Low AI processing latency is crucial to ensure smooth, responsive gameplay that adapts to the player's skill level without noticeable delays.
c. Data Pipeline Efficiency
Description: Measures the speed and reliability of data flow from collection to AI processing to implementation.
Example: A large e-commerce marketplace uses this KPI to ensure that their AI has access to up-to-date inventory, pricing, and user data. High data pipeline efficiency allows for real-time personalization and dynamic pricing strategies.
Conclusion: The Future of AI-Driven KPIs
As AI continues to evolve, so too will the KPIs we use to measure website success. Future developments may include:
- Emotional Response KPIs: Using computer vision and NLP to gauge users' emotional responses to website elements.
- Cross-Platform Journey KPIs: AI-powered tracking of user journeys across devices and platforms for a truly holistic view of performance.
- Predictive Trend KPIs: Metrics that don't just measure current performance but predict future trends and potential issues.
The key to success with AI-driven website optimization is not just in implementing the technology, but in effectively measuring its impact. By focusing on these KPIs, you can ensure that your AI investments are driving real, measurable improvements to your website's performance.