No-Code vs. Traditional Analytics: A Comprehensive Analysis of Capabilities, Costs, and ROI
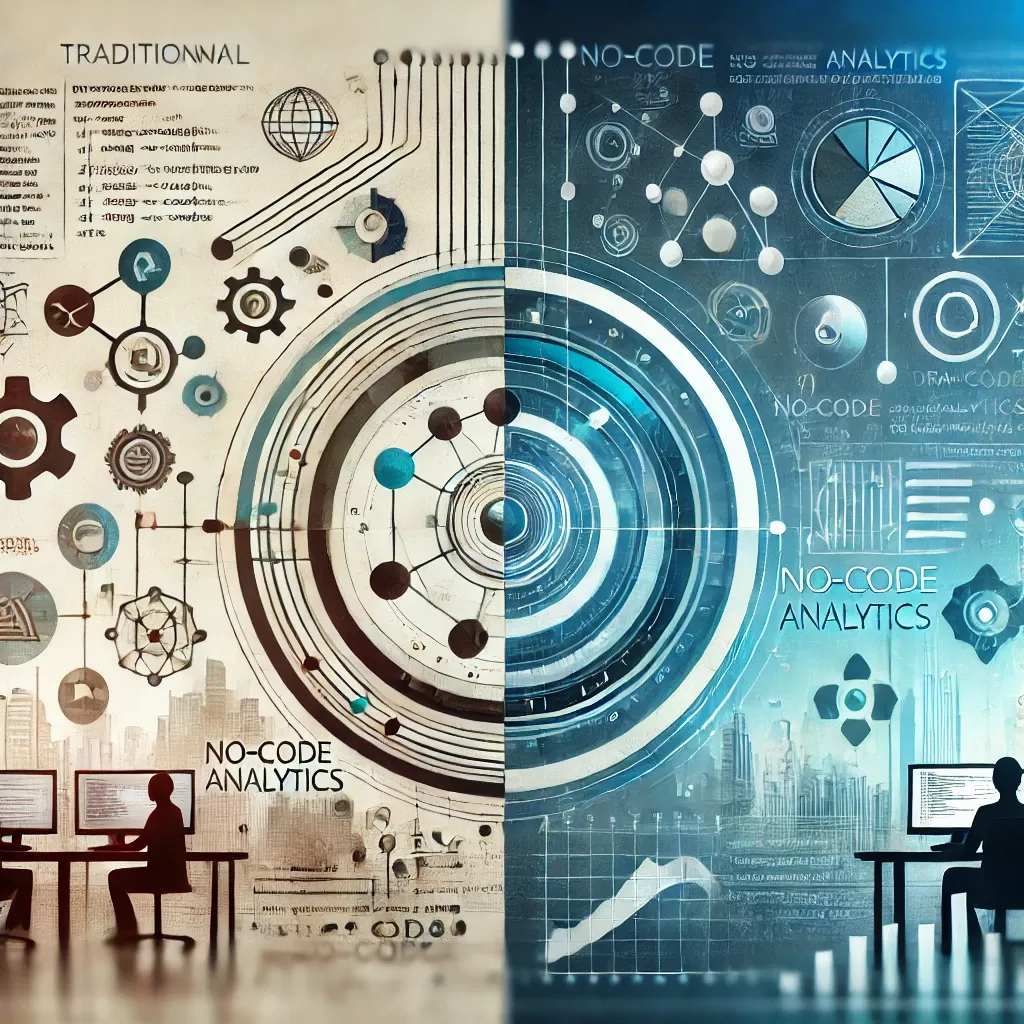
In the rapidly evolving landscape of data analytics, businesses face a crucial decision: should they stick with traditional analytics tools or make the switch to emerging no-code platforms? This comprehensive blog post will dive deep into the strengths and limitations of both approaches, helping you make an informed decision for your organization's needs. We'll also provide a detailed analysis of the costs involved and potential return on investment (ROI) for each approach.
Understanding the Landscape
Before we delve into the comparison, let's define our terms:
- Traditional Analytics Tools: These are established software solutions that often require coding skills and technical expertise. Examples include SAS, R, Python with libraries like Pandas, and SQL-based tools.
- No-Code Analytics Platforms: These are newer, user-friendly platforms that allow users to perform complex data analytics tasks without writing code. They often use visual interfaces and drag-and-drop functionalities.
Now, let's compare these two approaches across several key dimensions.
1. Ease of Use and Accessibility
Traditional Analytics
Strengths:
- Offers granular control over data processing and analysis
- Allows for highly customized and complex analyses
Limitations:
- Steep learning curve
- Requires coding skills and technical expertise
- Limited accessibility for non-technical users
No-Code Analytics
Strengths:
- User-friendly interfaces with drag-and-drop functionality
- Accessible to non-technical users
- Faster onboarding and training
Limitations:
- May not offer the same level of granular control as coding
- Some complex analyses might be challenging to implement
Real-world impact: A mid-size marketing firm switched to a no-code analytics platform and saw a 70% increase in the number of employees actively using data for decision-making within six months.
2. Flexibility and Customization
Traditional Analytics
Strengths:
- Highly flexible and customizable
- Can handle unique and complex analytical requirements
- Extensive libraries and packages available for various needs
Limitations:
- Customization often requires significant time and expertise
No-Code Analytics
Strengths:
- Pre-built templates and modules for common analyses
- Visual customization of reports and dashboards
Limitations:
- May have limitations for highly specialized or unique analytical needs
Real-world impact: A large e-commerce company uses a hybrid approach, employing no-code tools for 80% of their analytics needs, while maintaining a small team of data scientists for complex, custom analyses, optimizing both efficiency and depth of insight.
3. Scalability and Performance
Traditional Analytics
Strengths:
- Can handle very large datasets efficiently
- Allows for optimization of performance at a code level
Limitations:
- Scaling may require significant infrastructure investments
- Performance optimization requires specialized skills
No-Code Analytics
Strengths:
- Often cloud-based, offering easy scalability
- Automated performance optimization
Limitations:
- May have limitations with extremely large datasets
- Less control over performance optimization
Real-world impact: A fast-growing startup used a no-code analytics platform to scale their data operations from handling 100,000 to 10 million daily active users without needing to hire a dedicated data engineering team.
4. Integration and Data Connection
Traditional Analytics
Strengths:
- Can potentially connect to any data source with the right code
- Allows for custom ETL (Extract, Transform, Load) processes
Limitations:
- Setting up connections and ETL can be time-consuming
- Requires ongoing maintenance
No-Code Analytics
Strengths:
- Pre-built connectors for popular data sources
- Visual ETL builders
Limitations:
- May have limitations with uncommon or highly specialized data sources
Real-world impact: A healthcare provider was able to integrate data from 15 different systems using a no-code platform in just two months, a process that their IT department estimated would have taken over a year with traditional methods.
5. Security and Compliance
Traditional Analytics
Strengths:
- Allows for customized security implementations
- Can be run entirely on-premises if required
Limitations:
- Security depends heavily on the expertise of the implementers
- Keeping up with security best practices requires ongoing effort
No-Code Analytics
Strengths:
- Often comes with built-in security features
- Regular updates and patches managed by the vendor
Limitations:
- May not allow for highly customized security configurations
- Cloud-based solutions may not be suitable for all compliance requirements
Real-world impact: A financial services firm was able to implement a no-code analytics solution that met their strict regulatory requirements, with the vendor providing detailed compliance documentation that saved months of internal security review.
6. Future-Proofing and Innovation
Traditional Analytics
Strengths:
- Allows for implementation of cutting-edge techniques
- Not locked into a single vendor's innovation timeline
Limitations:
- Keeping up with latest trends requires continuous learning and updating
No-Code Analytics
Strengths:
- Regular updates with new features and capabilities
- Democratizes access to advanced analytics techniques
Limitations:
- Innovation pace depends on the platform provider
Real-world impact: A retail company using a no-code platform was able to implement AI-driven demand forecasting across their entire product line in weeks, a project they had been struggling to start with their traditional tools due to lack of AI expertise.
7. Cost Considerations and ROI
Understanding both the costs involved and the potential return on investment (ROI) is crucial when evaluating analytics solutions. Let's dive deeper into the financial aspects of both traditional and no-code analytics approaches.
Traditional Analytics
Costs:
- Licensing fees for proprietary software (e.g., SAS, Tableau)
- Hardware and infrastructure costs (servers, data warehouses)
- Salaries for skilled data scientists, analysts, and engineers
- Ongoing training and skill development
- Maintenance and updates
ROI Factors:
- Highly customized solutions can provide significant value if properly implemented
- Potential for deep, complex analyses that drive major business decisions
- Long-term value from developed intellectual property and in-house expertise
Potential ROI Scenario:
A large financial institution invested $2 million in a traditional analytics setup, including software licenses, hardware, and a team of five data scientists. Over three years, this team developed predictive models that improved fraud detection by 30%, saving the company an estimated $10 million in potential losses. ROI: 400% over three years.
No-Code Analytics
Costs:
- Subscription fees (often based on number of users or data volume)
- Initial setup and integration costs
- Training for staff (generally lower than for traditional analytics)
- Potential costs for premium features or increased data usage
ROI Factors:
- Faster time-to-insight, leading to quicker business value
- Broader adoption across the organization, fostering a data-driven culture
- Reduced need for specialized technical staff
- Scalability without significant additional investment
Potential ROI Scenario:
A mid-size e-commerce company switched to a no-code analytics platform, investing $100,000 in the first year (including subscription and training). They were able to reduce their data team from five specialists to two, saving $300,000 in salaries. Moreover, the broader access to data insights led to a 15% increase in customer retention, valued at $500,000. ROI: 700% in the first year.
Comparative Analysis
- Initial Investment:
- Traditional: Generally higher due to software licenses, hardware, and skilled personnel.
- No-Code: Usually lower, with costs spread out through subscription models.
- Ongoing Costs:
- Traditional: Can be significant, including maintenance, upgrades, and continued training.
- No-Code: More predictable, primarily consisting of subscription fees that may increase with usage.
- Scalability Costs:
- Traditional: Scaling up often requires significant additional investment in hardware and personnel.
- No-Code: Typically scales more cost-effectively, with incremental increases in subscription fees.
- Time to Value:
- Traditional: Longer time to value due to setup and development time, but potentially higher value for complex, unique needs.
- No-Code: Faster time to value, with out-of-the-box solutions providing quick insights.
- Risk:
- Traditional: Higher risk due to larger upfront investment, but potentially higher reward for successful implementations.
- No-Code: Lower risk due to lower initial investment and faster deployment, but potential limitations for highly specialized needs.
ROI Considerations
When calculating ROI for analytics solutions, consider these factors:
- Direct Cost Savings: Reduction in manual data processing, reporting time, and IT overhead.
- Indirect Cost Savings: Improved decision-making leading to operational efficiencies.
- Revenue Increases: Better insights leading to improved marketing, sales, and customer retention.
- Time Savings: Faster report generation and more efficient data analysis processes.
- Strategic Value: Long-term benefits of fostering a data-driven culture.
Real-world ROI Impact
A retail chain compared the ROI of their traditional analytics system with a new no-code platform:
Traditional System:
- Annual Cost: $500,000 (licenses, hardware, personnel)
- Annual Benefit: $750,000 (from data-driven decisions)
- ROI: 50%
No-Code Platform:
- Annual Cost: $200,000 (subscription, training)
- Annual Benefit: $1,000,000 (from wider adoption and faster insights)
- ROI: 400%
The no-code platform not only cost less but provided higher returns due to broader adoption across the organization and faster implementation of data-driven strategies.
Conclusion: Choosing the Right Approach
The choice between no-code and traditional analytics isn't always an either/or decision. Many organizations are finding success with a hybrid approach, using no-code platforms to democratize data access and insights across the organization, while maintaining some traditional analytics capabilities for specialized needs.
Consider your organization's specific needs:
- If you need to democratize data access and insights across your organization quickly, no-code platforms offer significant advantages.
- If you have highly specialized analytical needs and the technical expertise to support them, traditional analytics tools may still be necessary.
- For many organizations, a combination of both approaches may provide the best balance of accessibility, flexibility, and depth of analysis.
When evaluating options, consider both short-term costs and long-term value. No-code platforms typically offer faster time-to-value and lower upfront costs, making them an attractive option for many businesses. However, organizations with highly specialized analytics needs may find the investment in traditional analytics capabilities worthwhile.
Ultimately, the goal is to choose the approach that best enables your organization to make data-driven decisions efficiently and effectively. As the field of analytics continues to evolve, staying adaptable and open to new approaches will be key to maintaining a competitive edge.