The Future of Funnel Conversion: Machine Learning Insights
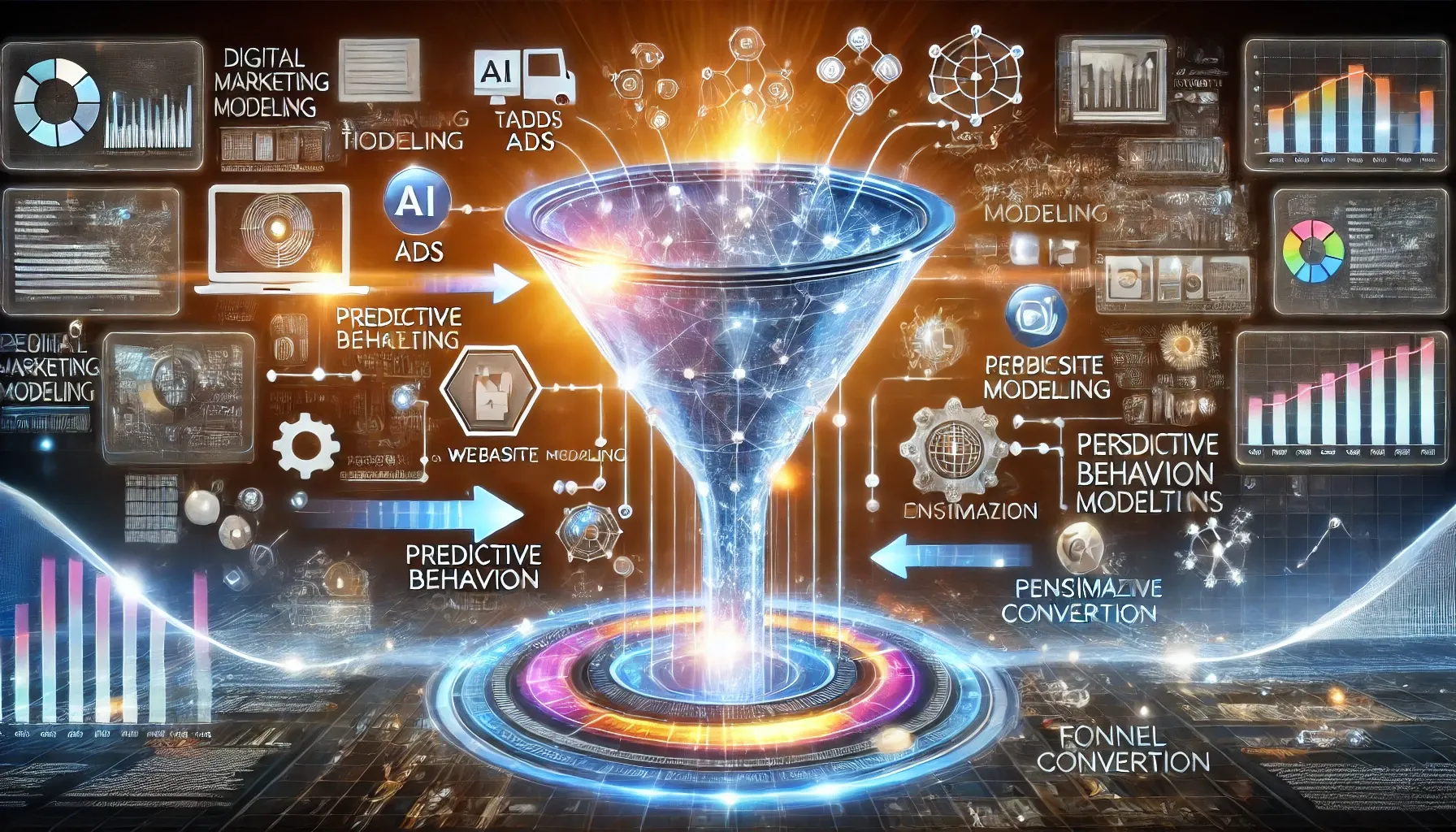
In the ever-evolving world of digital marketing, staying ahead of the curve is crucial for business success. One area that's seeing rapid transformation is funnel conversion optimization, thanks to the power of machine learning (ML). Let's dive into how ML is reshaping the future of funnel conversion and what insights it brings to the table.
Understanding the Funnel Through Machine Learning
Traditional funnel analysis often relies on static models and human interpretation. Machine learning, however, brings a dynamic and data-driven approach to understanding customer journeys. By analyzing vast amounts of user data, ML algorithms can identify patterns and behaviors that human analysts might miss.
Key Insights:
- Predictive Behavior Modeling: ML can predict which actions are likely to lead to conversions, allowing marketers to optimize their funnels proactively.
- Example: An e-commerce site uses ML to predict that customers who view product videos are 30% more likely to make a purchase, leading to strategic placement of video content throughout the funnel.
- Personalized Funnels: Instead of a one-size-fits-all approach, ML enables the creation of personalized funnels tailored to individual user behaviors and preferences.
- Implementation: A B2B software company uses ML to create dynamic landing pages that adjust content based on the visitor's industry, company size, and previous interactions with the site.
- Multi-touch Attribution: ML algorithms can more accurately attribute conversions across multiple touchpoints, providing a clearer picture of the customer journey.
- Case Study: A retail brand implements ML-driven attribution, revealing that social media interactions play a more significant role in conversions than previously thought, leading to a reallocation of marketing budget.
Real-Time Optimization
One of the most exciting aspects of ML in funnel conversion is its ability to optimize in real-time. As user behavior changes, so too can the funnel, adapting to maximize conversion chances.
How It Works:
- Continuous data collection and analysis
- Instant implementation of optimizations
- Constant learning and refinement of strategies
Real-World Applications:
- Dynamic Pricing: E-commerce platforms use ML to adjust prices in real-time based on demand, competitor pricing, and individual user behavior.
- Content Recommendations: News websites employ ML algorithms to suggest articles that are most likely to keep users engaged, reducing bounce rates and increasing ad revenue.
- Chatbot Optimization: Customer service chatbots use ML to continuously improve their responses based on user interactions, enhancing the support experience and guiding users through the funnel more effectively.
Uncovering Hidden Patterns
Machine learning excels at finding correlations and patterns in complex datasets. This capability is particularly valuable in funnel conversion, where subtle factors can significantly impact user decisions.
Examples:
- Identifying micro-conversions that lead to major conversions
- Recognizing seasonal trends and adjusting strategies accordingly
- Spotting unexpected barriers in the conversion process
Deep Dive: The Power of Micro-Conversions
ML algorithms have revealed that certain small actions, previously overlooked, can be strong indicators of future conversions. For instance:
- Newsletter signups correlating with higher lifetime customer value
- Product comparison tool usage leading to increased add-to-cart rates
- Social media sharing of products indicating higher purchase intent
By identifying these micro-conversions, businesses can optimize their funnels to encourage these behaviors and improve overall conversion rates.
Enhanced Customer Segmentation
Gone are the days of broad customer segments. ML allows for hyper-granular segmentation, enabling marketers to tailor their funnels to highly specific user groups.
Benefits:
- More targeted messaging and offers
- Improved allocation of marketing resources
- Higher conversion rates due to increased relevance
Advanced Segmentation Techniques:
- Behavioral Clustering: ML algorithms group users based on similar browsing and purchasing patterns, allowing for more nuanced targeting.
- Predictive Lifetime Value: Segmenting customers based on their predicted future value, enabling businesses to invest more in high-potential customers.
- Cross-Channel Segmentation: Integrating data from multiple channels (web, mobile, in-store) to create a holistic view of customer segments.
Predictive Analytics for Funnel Optimization
Machine learning's predictive capabilities are perhaps its most powerful feature for funnel conversion. By analyzing historical data, ML can forecast future trends and user behaviors.
Applications:
- Predicting which leads are most likely to convert
- Forecasting the impact of potential funnel changes
- Identifying the optimal timing for marketing interventions
Case Study: Predictive Lead Scoring
A SaaS company implemented an ML-driven lead scoring system that analyzed over 100 data points per lead. The system was able to:
- Predict with 85% accuracy which leads would convert within 30 days
- Identify the most effective nurturing strategies for different lead segments
- Reduce the sales cycle by 20% by focusing efforts on high-potential leads
Challenges and Considerations
While the future of funnel conversion with ML is bright, it's not without challenges:
- Data Privacy: As ML relies on user data, ensuring compliance with privacy regulations is crucial.
- Solution: Implement robust data anonymization techniques and transparent opt-in processes for data collection.
- Interpretability: Some ML models can be "black boxes," making it difficult to understand their decision-making process.
- Approach: Utilize explainable AI techniques to provide insights into model decisions, helping marketers understand and trust the recommendations.
- Over-optimization: There's a risk of creating funnels that are too rigid, potentially missing out on unexpected opportunities.
- Mitigation: Maintain a balance between ML-driven optimization and human intuition. Regularly test new ideas alongside ML recommendations.
- Data Quality and Quantity: ML models are only as good as the data they're trained on.
- Strategy: Invest in data collection and cleaning processes. Consider augmenting first-party data with carefully selected third-party data sources.
The Future of ML in Funnel Conversion
Looking ahead, several emerging trends are set to further revolutionize funnel conversion:
- AI-Powered Copywriting: ML models will generate and test multiple versions of ad copy, email subject lines, and product descriptions in real-time.
- Voice and Visual Search Optimization: As these technologies grow, ML will play a crucial role in optimizing funnels for voice commands and image-based searches.
- Emotion AI: Advanced ML models will analyze user emotions through facial expressions, voice tonality, and text sentiment, allowing for emotionally intelligent funnel optimization.
- Cross-Platform Journey Optimization: ML will enable seamless funnel experiences across devices and platforms, creating a truly omnichannel conversion strategy.
Conclusion
Machine learning is set to revolutionize funnel conversion optimization. By providing deeper insights, enabling real-time adaptations, and offering predictive capabilities, ML is empowering marketers to create more effective, efficient, and personalized conversion funnels.
As we look to the future, businesses that embrace these ML-driven insights will likely see significant improvements in their conversion rates and overall marketing effectiveness. The key will be to balance the power of ML with human creativity and strategic thinking, creating conversion funnels that are both data-driven and user-centric.
The future of funnel conversion is not just about collecting more data, but about extracting actionable insights from that data. Machine learning is the tool that will allow marketers to do this at scale, opening up new possibilities for personalization, optimization, and ultimately, business growth.